Enterprise Model Inventory
Establish a centralized inventory of all your model assets
Organizations use Verta to create a single, searchable model catalog, enabling robust AI governance, end-to-end ML lifecycle management and accelerated value realization
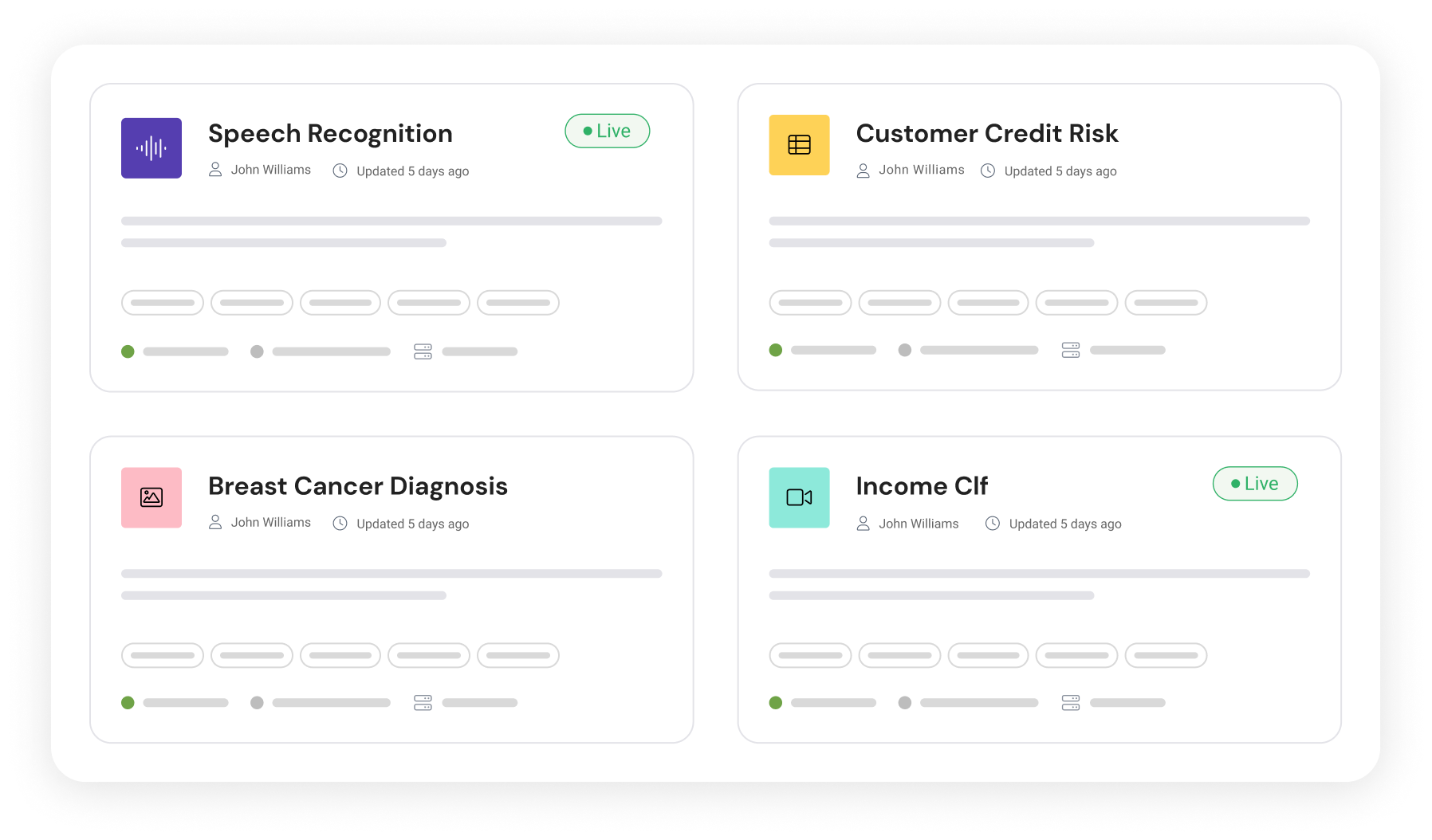
What Verta can solve
Organizations can't effectively govern their AI portfolios if they don't know what models they have in production and where those models are being used.
The typical annual attestations can't keep up with AI/ML innovation, and data scientists wind up duplicating effort because they have no visibility into current assets and past work. To deliver optimal value from their ML investments, organizations first must bring all their ML assets into a single, centralized model inventory.
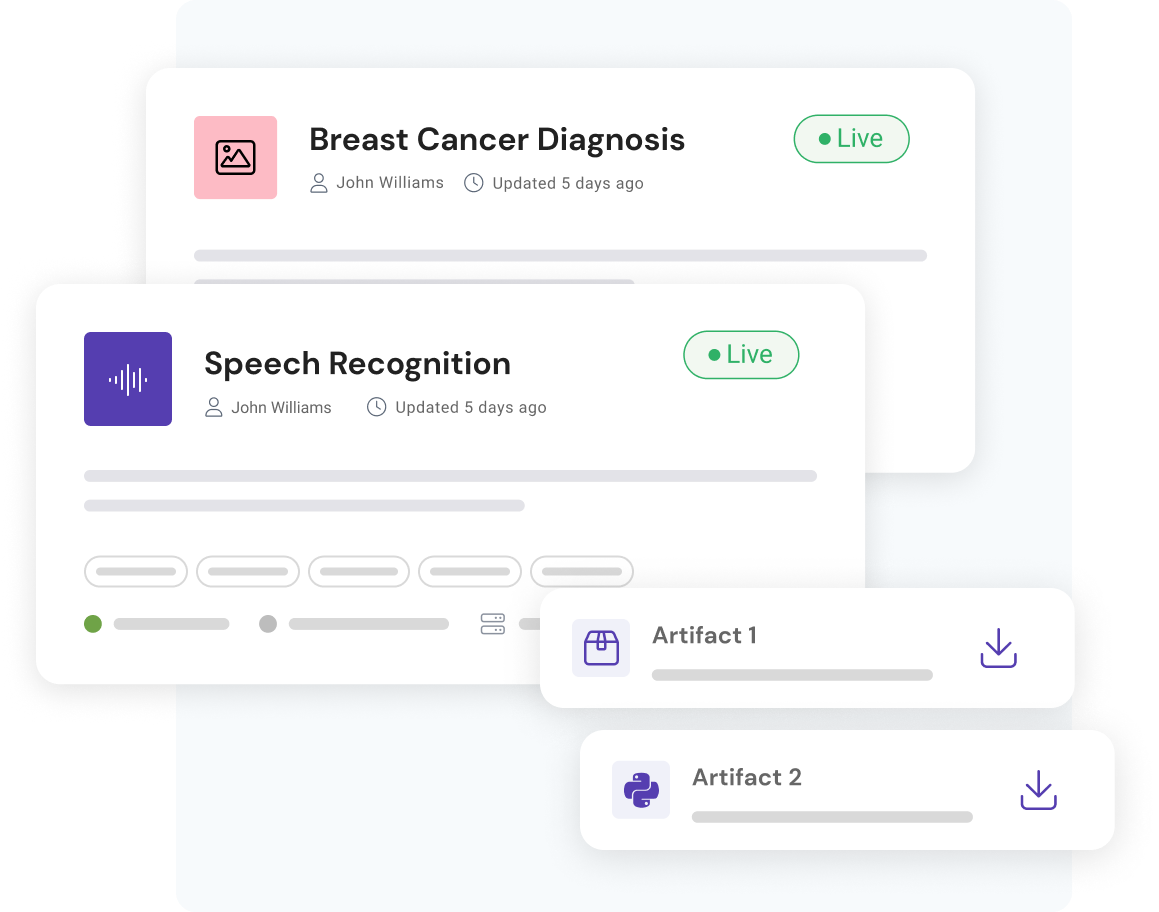
Make model discovery, integration, governance and reuse easy a with real-time, always up-to-date inventory
Publish all model metadata, documentation and artifacts in one central catalog
Find up-to-date model documentation, API contracts and container packaging, and stay current with changes
Centrally monitor model I/O and performance in real time, and administrate governance rules
Track adoption and growth of AI/ML programs throughout the enterprise, and discover opportunities to reuse existing assets
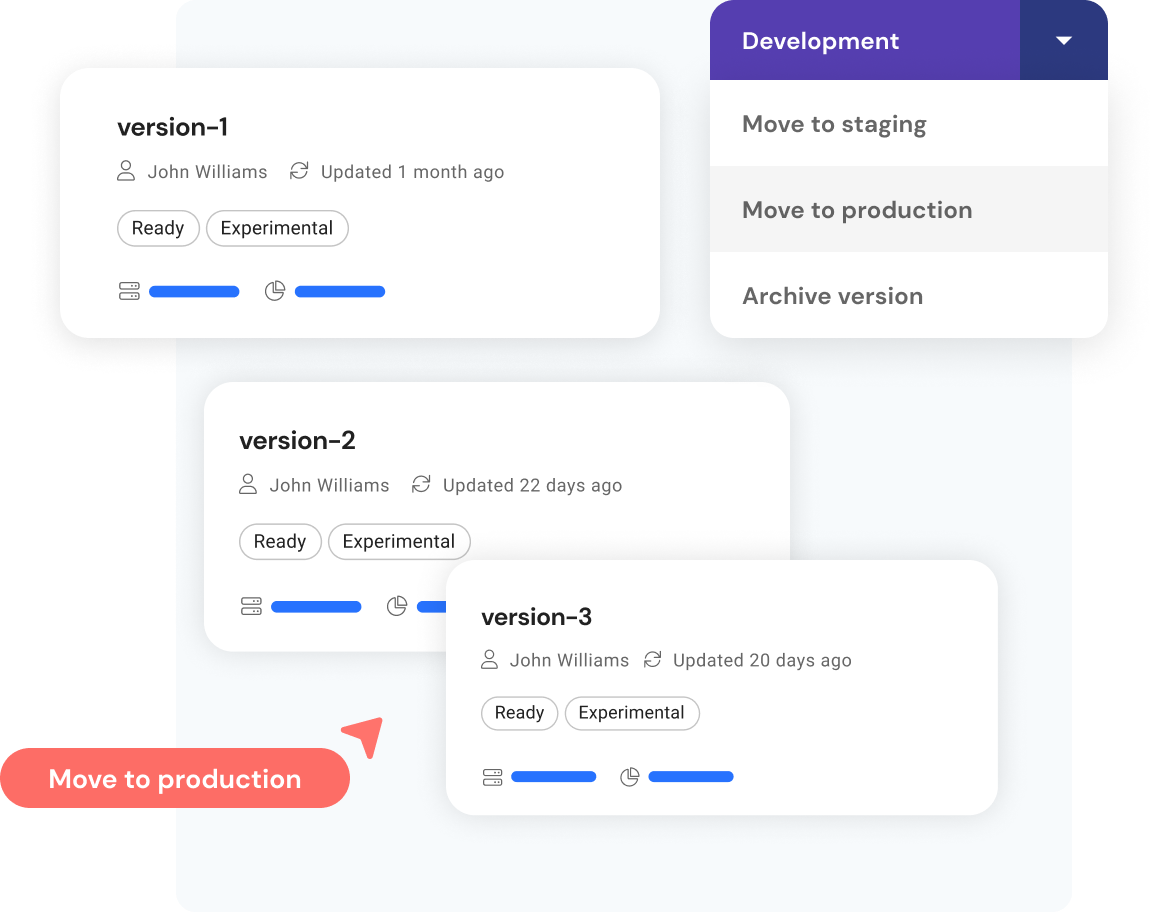
Record and manage release lifecycles from development and staging to production and archive
Automatically track model versions to monitor the progress of ML iterations and simplify the model update process
Ensure models are ready for production release with custom governance checklists
Scan models for vulnerabilities and review training data for bias easily
Use web hooks to trigger downstream actions for model validation and deployment
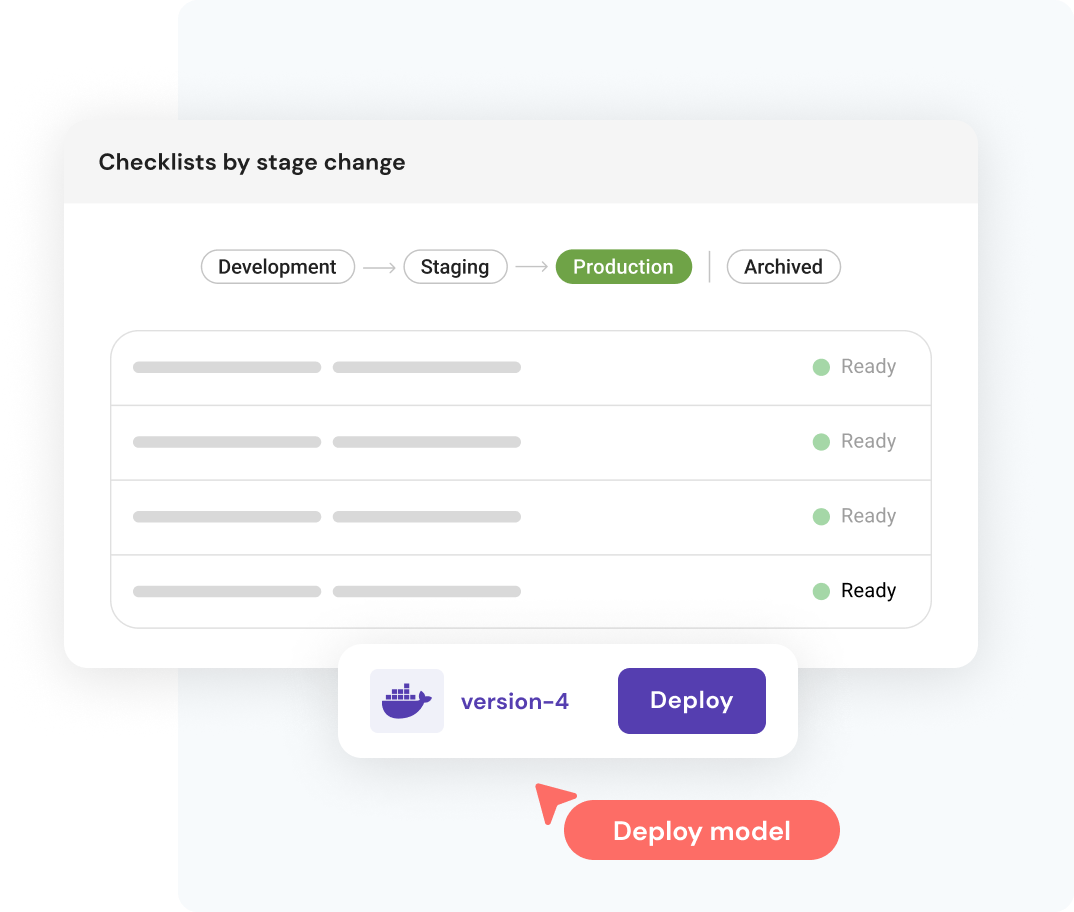
Deploy models confidently with easy-to-use but powerful governance tools, and monitor model performance throughout the ML lifecycle
Gain enterprise-wide visibility and transparency to your organization’s assets in use, retired and on deck, including on-prem and external deployments
Easily configure deployments and monitor all models in production within the platform
Customize and configure promotion and deployment checklists
Standardize model documentation, model schema and API management across your ML assets
Access detailed audit logs for compliance
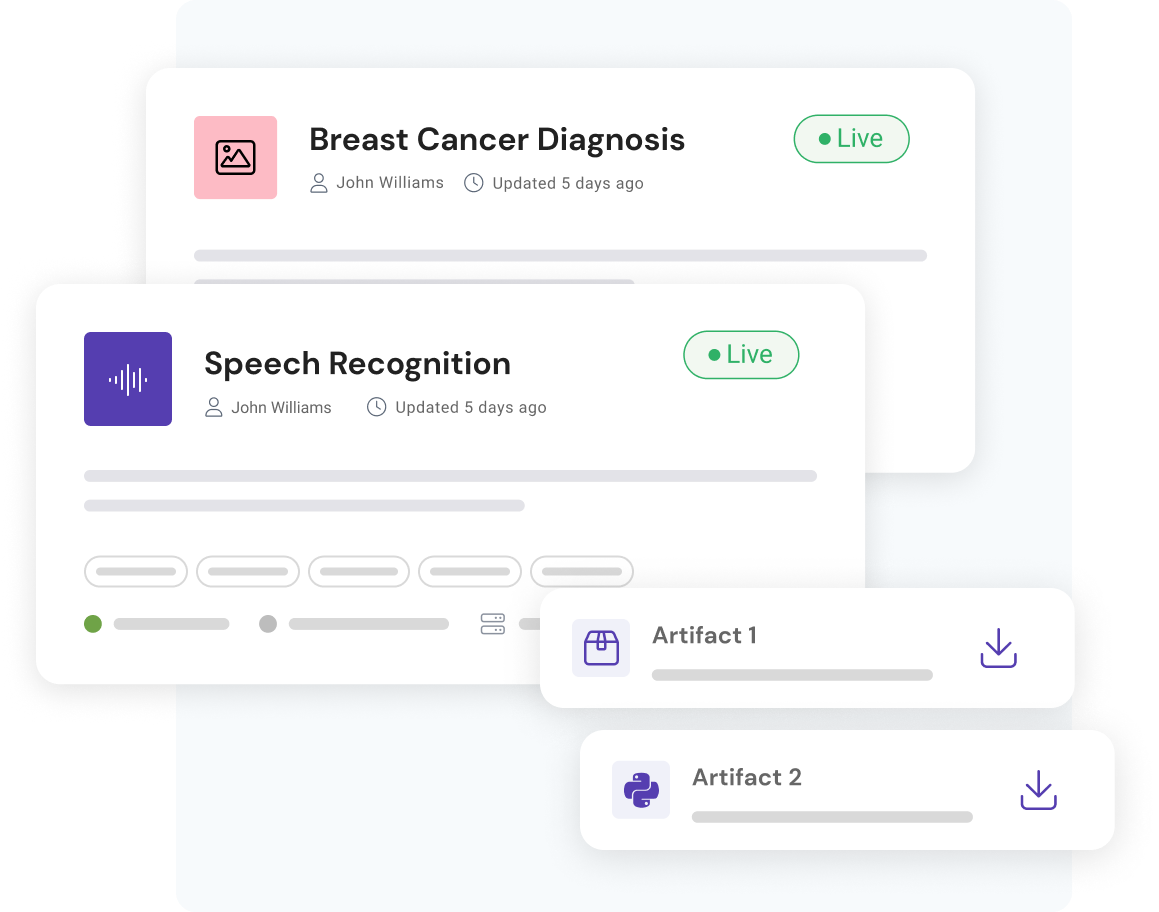
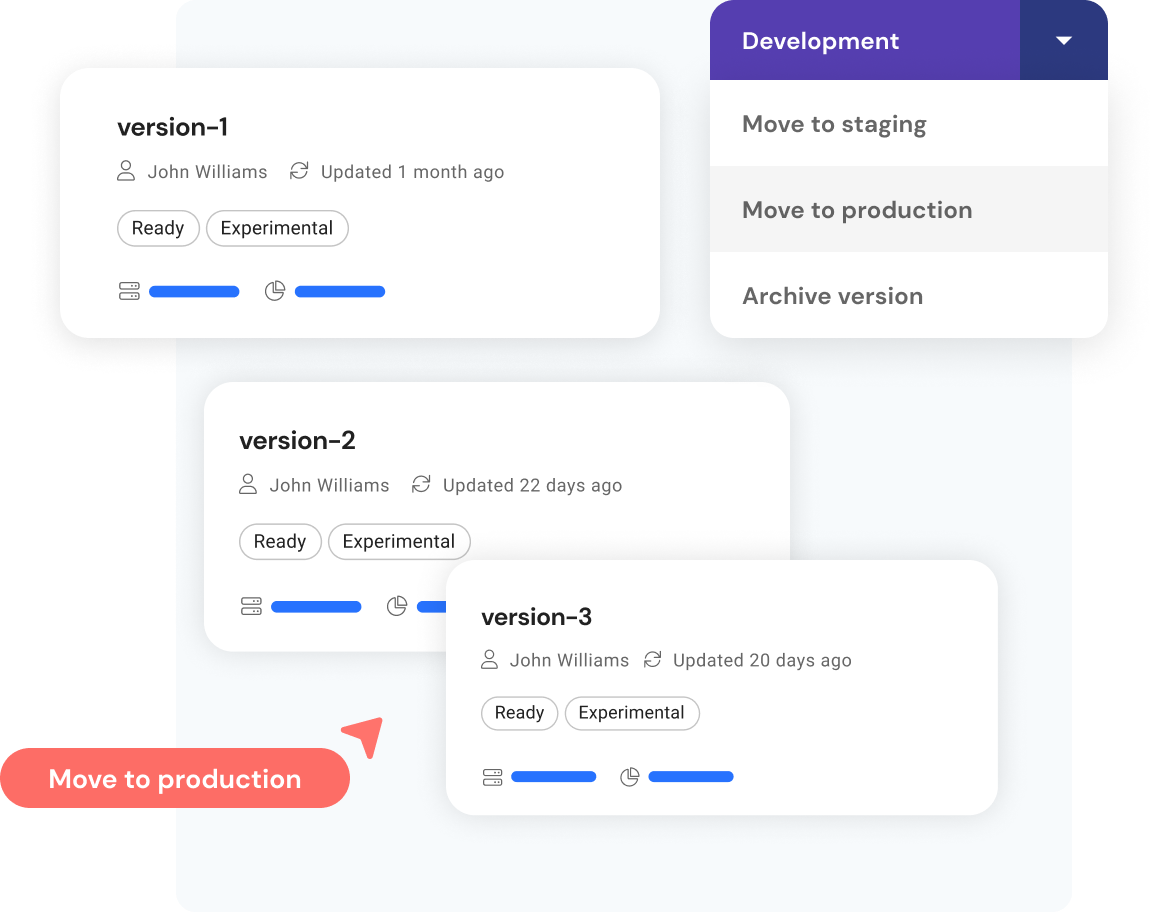
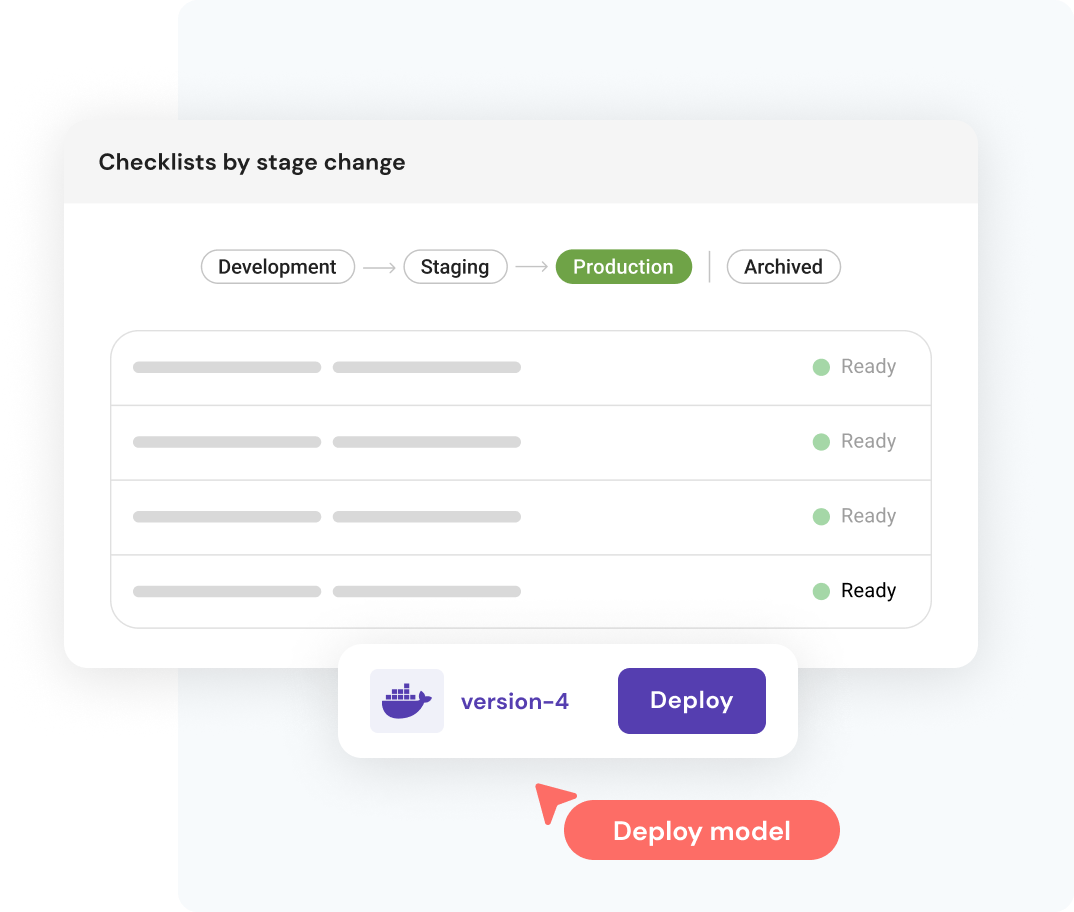